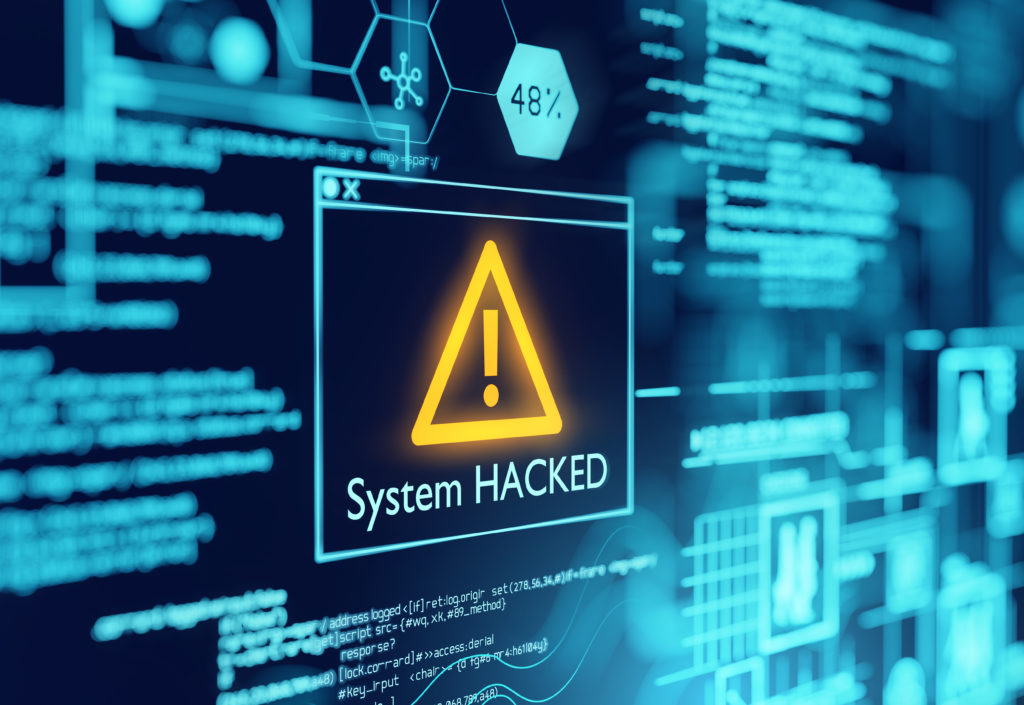
Periodo
1-SEPT-2021 a 31-AGO-2024
Detección de ciberataques en “industria conectada” e IoT mediante integración y correlación de alertas multifuente (COINCYDE)
Referencia
PID2020-115199RB-I00
Organismos / empresas
Ministerio de Ciencia e Innovación
Investigadores
- Jesús E. Díaz Verdejo - IP
- Juan Carlos Cubero Talavera - IP
- Francisco Cortijo Bon
- Antonio Estepa Alonso
- Rafael Estepa Alonso
- Germán Madinabeitia Luque
- Olga Pons Capote
- Amparo Vila Miranda (baja por jubilación)
Equipo de trabajo
- Vicente Mayor Gallego
- Agustín W. Lara
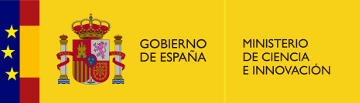
Este proyecto está financiado por MCIN/ AEI/10.13039/501100011033/
Resumen
Los sistemas de monitorización de la seguridad en red (NSM) se encuentran hoy en día entre los componentes más relevantes para la detección y respuesta a los ciberataques. Sin embargo, sus capacidades de detección se limitan en su mayoría a ataques conocidos y tienden a generar una gran cantidad de alertas, muchas de las cuales son falsos positivos. Así, los operadores de ciberseguridad (CSO) deben supervisar una gran cantidad de alertas para determinar la ocurrencia real de incidentes, mientras que algunos de ellos permanecen sin ser detectados. Este proyecto tiene como objetivo desarrollar nuevas técnicas para mejorar las capacidades de detección mediante la adición de nuevos métodos basados en anomalías combinados con la correlación y priorización de alertas incorporando información contextual de la red. Esto mejorará la calidad de las alertas y reducirá la tasa de falsos positivos.
En esta propuesta se plantea el desarrollo de un NSM específico para plantas industriales con elementos del Internet of Things (IoT) y, más concretamente en uno de sus usos verticales: las SmartCity. Las instalaciones que pueden beneficiarse de la solución objeto de este proyecto son aquellas que permiten el control y monitorización de parques de dispositivos inteligentes (IoT, SmartCity), desde una aplicación o servicio web que se utiliza como interfaz de usuario para la gestión de servicios inteligentes. La elección del escenario tiene una triple motivación. Primero, por la gran relevancia y expansión de este tipo de redes en la actualidad. Segundo, el escenario plantea una serie dificultades y requisitos específicos que no han sido convenientemente abordados en los SIEM actuales. Y tercero, la selección del escenario permite acotar el contexto, lo que posibilita un abordaje adecuado de la incorporación de información contextual.
El sistema a desarrollar incorporará múltiples detectores, incluyendo los usados habitualmente, considerando nuevos detectores específicos para el escenario que están orientados a las diversas amenazas existentes. Así, se desarrollarán detectores basados en anomalías a nivel del tráfico observado (flujos), a nivel de aplicación (sensorización) y a nivel de los servicios web usados para la operación remota. Adicionalmente, se hará uso de técnicas de inteligencia artificial para la correlación y priorización de las alertas incorporando información relativa al estado e historia previa de la red. Esto permitirá identificar falsos positivos, reducir el número de alertas finalmente enviadas al CSO y mejorar la información en las mismas.
Un elemento relevante y novedoso es el uso de una matriz de tráfico generada a partir de flujos en diferentes escalas de tiempo. Esta matriz contiene información sobre las conexiones de red que pueden explotarse para múltiples usos. Así, se pueden establecer algunos indicadores de compromiso para identificar ataques. También se puede utilizar para aplicar varios tipos de análisis de minería de datos, como la búsqueda de patrones comunes entre flujos, realizar perfiles de tráfico de servicios, evaluar la importancia y encontrar relaciones entre activos. La información extraída de esta matriz se utilizará como información contextual en la correlación y priorización de alertas.
Finalmente, la arquitectura propuesta incluye realimentación a partir de las acciones del CSO, lo que permite evaluar la calidad de detección y priorización y ajustar el rendimiento del sistema.
Antecedentes
Network infrastructure has become a critical asset in nowadays organizations as it enables information exchange between user terminals and corporate servers (either locally hosted or in the Internet). Network downtimes can have a tremendous impact in productivity and reputation. Cyberattacks are increasingly frequent and with more relevant effects, as shown in the famous case of “Wannacry” ransomware attack with more than 300 000 devices affected in 166 different countries. New cases are yet to come with unprecedented impact, potentially affecting critical infrastructure such as hospitals, facilities, industry, etc.
The evolution towards the Internet of Things (IoT) and the incorporation of new network-based control and monitoring systems in the so-called Connected Industry, put the focus on the need to protect these systems from cyberattacks.
Organizations address cybersecurity with a combination of tools, procedures and practices. Most organizations deploy monitoring systems —typically called Network Security Monitoring (NSM), or Security Information and Event Management (SIEM) [1] — to detect and react against attacks. These systems process heterogeneous information from multiple sources such as traffic flows seen by network elements, alerts generated by Intrusion Detection Systems (IDS) [2] or event logs from services. The large amount of information to be processed has become one of the main challenges to properly prioritize and classify alerts in real time.
Although many, even commercial, NSMs exist, they still lack of many desirable properties regarding the detection capabilities and the quality and the volume of alerts they generate, requiring the supervision from a human expert (the Cybersecurity Officer, CSO). Thus, a key issue regarding NSMs is to improve its performance in terms of the attacks they are able to discover and the reduction of the number of non-relevant alerts (false positives).
The motivation above justifies the development of new techniques allowing to improve alert generation and prioritization in a timely manner, integrating and correlating the information from the multiple available sensors and detectors. This is especially relevant in the IoT and connected industries scenarios, as the number of field elements can be really high and the impact of an attack or malfunction can be enormous, especially in the case of critical infrastructures as a power plant. Furthermore, industrial and IoT scenarios poses specific characteristics (see later in this section) that makes the detection even more defying, while no specific NSM is available.
Thus, the context of this project includes a scenario with multiple data sources, which generate a high volume of information potentially relevant to the modeling and prioritization of security incidents. This is the right context for the application of data mining techniques that enable the generation of knowledge. The application of such techniques could produce a novel and significant advance in the field of NMS because it would improve detection capabilities and the understanding of incidents by establishing new relations among information coming from different data sources. On the other hand, these techniques can improve the characterization of events that can be associated with attacks and/or can provide context information that enhances detection.
In sum, this project aims to improve the state of art in the context of IoT and Industrial plants in the following cybersecurity challenges identified in [3]:
-
- Reducing response time during incidents through the improvement of detection capabilities and the reduction of false positives. We propose methods to improve the detection of cyberattacks thanks to the correlation of the information from different sources and detectors.
- Identification and characterization of context-related events that, although unrelated to attacks, produce information that can be relevant to improve the detection and modeling of some cybersecurity incidents.
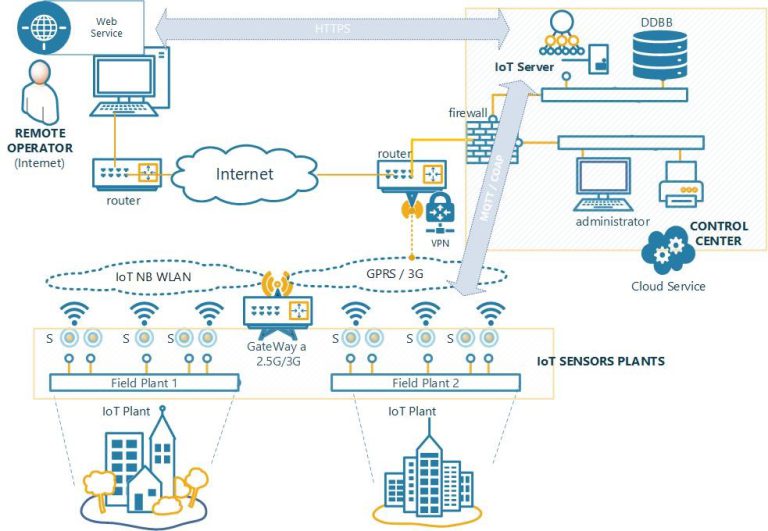
More precisely, this project aims at the development of a specific cybersecurity solution for industrial plants (Industrial Control Systems or ICS) with elements of the IoT and, more specifically, in one of its vertical uses: the SmartCity. The target is to develop a NSM able to provide significant information about on-going incidents by applying different intrusion detection approaches, including novel ones, to generate alerts that will be analyzed and correlated using IA-based techniques incorporating context information obtained from different sources and sensors across the installation. The facilities that can benefit from the solution object of this project are those that allow the control and monitoring of smart device parks (IoT, SmartCity), from an application or Web service that is used as a user interface for the management of intelligent services. To illustrate it, Fig. 1 shows the schematic of a typical Smart City installation for the intelligent control of lighting in multiple cities. This deployment will be used as the example scenario for the current project.
The IoT sensor plant in Fig. 1 is composed of 2 plants or field networks with sensors (called S in the figure) that correspond to intelligent lighting points remotely controlled from a management system (IoT Server in the figure) that is accessed through a Web application that allows remote management and operation of one or more plants. The Control Center, often located in the cloud, consists of several nodes and databases (DDBB in the figure) that store all the information of the system. The IoT Server is in turn responsible for the communication with the IoT nodes using the usual application protocols in IoT (e.g. MQTT or CoAP). The operation of the system is carried out from the Internet by the managing entity of the plants and includes the management of the IoT nodes (e.g., provision of procedures or operating points), and is carried out through a Web browser that uses a secure protocol (https with TLS 1.2 or higher). The access networks used by the IoT nodes can be private (e.g. Lora, Sigfox, NBWLAN networks with an Internet gateway / operator) or subcontracted to a network operator (e.g. GPRS or 3G), and communicate with the Control Center through a virtual private network (VPN) usually offered by the network operator.
It is worth mentioning the significant specificities of this scenario regarding the deployment of a “conventional” NSM system:
-
- Low throughput on the access data link to the IoT nodes: 2.5G coverage in many cases, and even lower data throughputs (e.g. SigFox, Lora).
- Remote operation of the installation: the Control Center is protected with a VPN provided by the network operator, but access to the IoT server is usually offered through the Internet with a username and password (so as not to be inconvenient for the operator or manager of the plants) against an https application.
- Use of IoT applications and protocols that are usually implemented with a low level of security (i.e. without communication encryption).
- The possibility of obtaining the same behavior pattern between 2 similar and geographically close intelligent lighting plants is peculiar, some application variables (e.g., instantaneous power consumed) should have a synchronous behavior.
Connected Industry (<i «>Industry 4.0), in addition to the usual cyberthreats of an IT network (e.g., physical access control, user/permission control, authentication policies -passwords-, etc.), we can highlight the following cyber threats particular to the scenario shown in the figure:
-
- Impersonation of the web session or identity of the plant operator
- Attack on the management application web server / application or the VPN server to operate the IoT infrastructure.</li
- Attacks that are difficult to detect by conventional intrusion detection equipment (IDS, Intrusion Detection Systems – it could be an application-level firewall). This type of attack would be known as: 0-day and APT (Advanced Persistent Threat) for the control of systems and theft of sensitive information.
- Attacks on IoT devices and infrastructure in the field network.
Due to the mentioned peculiarities, the possible commercial solutions for the early detection of cyberattacks in remotely managed industrial plants / IoT have differential characteristics compared to conventional cybersecurity systems (oriented to IT –Information Technology-) and should meet the following requirements:
-
- REQ1: Do not affect the normal operation of the equipment installed in the plant. This implies: using only passive security tools (that do not inject traffic) and a minimum consumption of the network bandwidth available at the installation.
- REQ2: Not significantly affect the cost of the installation. For this, in addition to the price associated with the acquisition and deployment of the cybersecurity system, the solution must have a low consumption of computational, storage, and network resources, ideally being able to be integrated into existing plant equipment as a virtual machine.
- REQ3: Wide detection capacity. The solution must cover the detection of significant security events linked to the threats described above, both existing and 0-day, as well as allow compliance with the applicable regulations and policies in each case.
[1] | Sanders, C.; Smith, J.; Applied Network Security Monitoring”, Syngress, (2014). ISBN: 978-0-12-417208-1. |
[2] | García-Teodoro, P.; Díaz-Verdejo, J.E.; y otros; “Anomaly-based Network Intrusion Detection: Techniques, Systems and Challenges”, Computers & Security, 28:18-28 (2009). |
[3] | Zuech, R.; Khoshgoftaar, T.; Wald, R.; “Intrusion detection and big heterogeneous data: a survey”, Journal of Big Data, 2:3 (2015). |
[4] | M. Kaouk, et.al.; «A Review of Intrusion Detection Systems for Industrial Control Systems», In Proc. 6th Int. Conf. on Control, Decision and Inf. Technologies, 1699-1704. |
[5] | Lu, Yang, and Li Da Xu; «Internet of things (IOT) cybersecurity research: A review of current research topics», IEEE Internet of Things Journal 6.2: 2103-2115 (2018). |
[6] | Sridhar, et.al.; “Model-based attack detection and mitigation for automatic generation control”, IEEE Transactions on Smart Grid, 5(2)580–591 (2014). |
[7] | Zhu, B., Joseph, A., & Sastry, S.; “A taxonomy of cyber attacks on SCADA systems”, In Proc. IEEE Int. Conf. on Internet of Things and Cyber, Physical and Social Computing. (2011). |
[8] | Kallitsis, M. G., Michailidis, G., & Tout, S.; “Correlative monitoring for detection of false data injection attacks in smart grids”, In proc. 2015 IEEE Int. Conf. on Smart Grid Communications, 386–391 (2016). |
Objetivos
The main objective of the project is the design and implementation of an innovative system for the early detection of cyber attacks specific for remotely managed industrial IoT environments. Thus the objective is to develop an IoT and connected industries oriented NSM able to provide significant information about on-going incidents by correlating the alerts from different existing and novel intrusion detection approaches using context information from different sources and sensors through the incorporation of data mining techniques in order to improve the quality of the detection in attack scenarios or failures (incidents).
The implementation includes a test pilot that allows to validate or readjust the design in a real plant, based on a final battery of tests on which to measure the performance and consumption of resources of the provided solution. To be suitable for commercial exploitation, this security solution must meet the following partial objectives/capabilities:
O1. Passiveness: The NSM must be passive so as not to affect existing systems.
O2. Detection capabilities: The NSM must offer a broad capacity to detect threats of various types, e.g. it must incorporate existing knowledge of defined known attacks, and must also incorporate the ability to detect 0-day attacks and APTs through the analysis of anomalies both at the traffic level and at the IoT application level.
O3. Multi-plant correlation: In the case of multi-plant installations, the system will apply spatio-temporal correlation techniques among similar plants to identify anomalous behaviors.
O4. Identity compromise detection: To detect spoofing attacks or the theft of credentials, anomalies will also be sought in the pattern of actions carried out by the users in the operation of the IoT plant.
O5. Integration: The NSM must be integrated into the operations console of an event management system in the operations center, offering information on cyberattacks to the operators of the IoT system. They will be able to take corrective actions and provide feedback to the detection system in order to minimize the false positive rate and bring the system to an optimal point of operation.
O6. Contextual information: The system must apply data mining and IA-based methods to correlate the alerts from the existing detectors, logs and sensors using contextual information in order to reduce the number of alerts sent to the administration console (by grouping the information related to the same incident) and to improve the detection and false positives rates.
The purpose of this proposal is twice. First, it pretends to advance in the scientific knowledge by the development of a novel integrated system suit for the considered scenario. This system will combine the adaptation of existing techniques, especially signature based ones, and the development and tuning of new ones. In particular, the proposal will integrate the concepts of context-aware detection, per-user behavior analysis and spatio-temporal correlation of alerts from similar plants into the anomaly detection process in an approach to identify specific threats for this kind of scenarios and to improve the detection capabilities. Second, it pretends to develop and evaluate a near-to-market prototype system that could be of interest for its incorporation into a catalogue of IoT cybersecurity solutions. This system will fill a gap that is currently available on the market.
To this end, a TRL6 demonstrator pilot of a cyberattack detection system in connected industry plants in multi-plant IoT environments will be developed.
Propuesta
Although the design of the solution is part of the project, it is possible to start from a simple block diagram that serves as a starting point and helps to structure and plan the proposal. The preliminary proposed solution (Fig. 2) integrates techniques to improve the detection capacity and performance in cybersecurity systems in the considered scenario. It aims to detect, classify and prioritize the incidents by considering different target oriented detectors and the holistic analysis of all the security related events through a context-aware analysis. It is composed of six main modules:
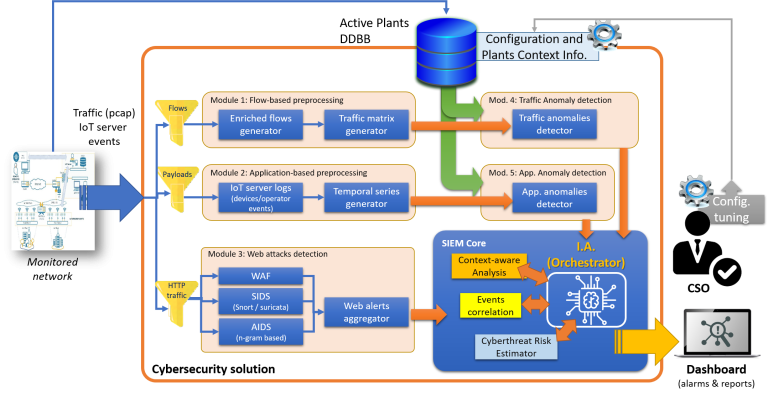
– Module 1: Flow-based Preprocessing. The target of this module is to generate the “traffic matrix” including information from all the observed flows after applying deep packet inspection techniques for its characterization.
– Module 2: Application-based Preprocessing. This module considers the communication from the field plants to the IoT server and extract the times series for the different monitored parameters and variables.
– Module 3: Web Attacks Detection. This module generates alerts related to the existing web-servers. It must include both signature-based methods and anomaly-based analysis in order to detect previously known attacks, 0-day and APT.
– Module 4: Traffic Anomaly Detection. Its target is to generate alerts from the analysis of the traffic matrix by using anomaly-based detection methods, i.e., it is oriented to the detection of anomalies in the traffic at flows level.
– Module 5: Application Anomaly Detection. This module looks for anomalies in the behavior of the elements in the field plant at the application level, i.e., it considers the evolution of the values of the monitored variables and parameters.
– Module 6: SIEM Core. It will process all the alerts from the different modules in order to correlate them and include contextual information in the analysis. This module will generate the per-incident alerts that will be presented to the CSO after filtering out those finally classified as false positives.
Modules 1 and 2 are preprocessing modules that extract the information needed by other modules. Modules 3, 4 and 5 are detector modules that generate raw alerts, each of them focused in a different feature/analysis. Finally, module 6 is the core of the system, as it will combine the information from the raw alerts and it will analyze them considering contextual information gathered by different techniques.
Thus, the overall operation of the system is as follows (Fig. 2). The solution must monitor the traffic flow seen by the IoT Server, as well as the most significant events from the application server —e.g., a log with user control actions, alarms, connections made, etc.— and the HTTP traffic to the web server. This information is fed into 3 different modules targeted at different kinds of analysis. The first two modules (modules 1 and 2) are preprocessing modules that extract and enrich the information associated to the observed flows and the payloads related to the IoT application. Thus, module 1 generates a traffic matrix after processing enriched flows through deep packet inspection techniques. Module 2 generates time series of IoT application events. The outputs from these modules together with the http payloads will fed, respectively, three different detectors (modules 3, 4 and 5) that will generate alerts associated to each of the different dimensions considered in the system. Finally, those alerts are feed to the SIEM core (module 6) for its further processing and the generation of the alerts that will be sent to the dashboard. The target of the SIEM core module is to enhance the detection capabilities by applying different sources of knowledge whose final objective is to improve CSO situational awareness. For this, the alerts will be correlated and prioritized having into account the information about the overall state of the system (context-aware analysis), the behavior of the operators and the relationships among the different generated alerts.
A feedback procedure is also considered. The CSO can apply a fine tuning of the system in order to reduce false positives or irrelevant alerts. For this, some parameters related to the global operation (i.e. equipment to filter out, thresholds for anomaly-based detectors, alarm filtering) will be considered.
From a threat point of view, the output of Module 1 makes it possible to find anomalies at the level of network traffic (Module 4), which makes it possible to easily detect scanning attacks, DDoS, etc. The output of Module 2 will allow to detect anomalies (Module 5) at application or plant level by looking for behavioral anomalies that could correspond to errors or operational problems or cyberattacks. Said anomalies can come both from the comparison of the time series with a self-learned normality pattern, as well as from the spatio-temporal correlation of this series with other series that could be correlated (e.g., light level detected with time of day, or light level detected by the IoT node with the light level detected by another IoT node in nearby location). This second correlation is especially interesting in the case of multi-site IoT systems. Finally, threats to the web server, i.e. web-based attacks, are handled by Module 3, that combines state of the art detectors with different capabilities. On top of these, SIEM core will provide an additional layer able to discriminate false positives and true positives by analyzing the relationships among events and the state of the overall system. As a simplistic example, an anomaly detected at the time series analysis —i.e. lights turned on at daylight— can derive from a legitimate order sent from the console —i.e. due to maintenance activities— and as such, should be labeled as a false positive.
From a research point of view, as previously mentioned, the proposed architecture try to merge well-known techniques and methods with novel proposals in order to provide an effective and practical NSM for the considered scenario. Some of the existing techniques should be adapted and optimized while others are to be explored in this context which will unquestionably represent contributions in the field. On the other hand, the correlation of security events and the reduction of the false positives rate constitutes a relevant challenge that has not yet been satisfactorily solved and that is the focus of current research. In this regard, major contributions are expected from the anomaly-based detection techniques as well as from the application of IA-related methods for the post-processing of the alerts.
Thus, the objective of the SIEM core module is the extraction of intelligence to determine the existence of certain relevant events from the point of view of security and the relationship among various data for its use in alerts analysis. Therefore, the objective is to develop methods that allow the extraction of intelligence for their use in the SIEM and to determine the parameters or significant data for the characterization of security related events. For this, at least the following methods will be considered:
– Frequent patterns extraction: Its objective is to determine the possible relationships between the data of the different sensors/elements. As an example, and in relation to the traffic matrix, it intends to establish the patterns of frequent interconnections (flows) among the assets.
– User/service profiles: Its objective is to establish profiles associated with the different types of users (e.g. plant operator, end user, administrative) and services. The output of this module is especially relevant for the evaluation of the impact of the incidents.
– Prediction of links/flows: This module will provide indicators regarding the probabilities of establishing new relationships (prediction) from a given state of the network. These probabilities are of interest both for the modelling of the attacks and for the prediction of risks.
– Outliers detection and clustering: Various methods will be applied for the detection of outliers in the state/characterization of the network, that is, for assessing the normality of an observed context. Similarly, clustering-based techniques will be applied both to stablish the context and to correlate observed alerts.
– Case-based reasoning: Case-based reasoning techniques will be applied, together with Mitre ATT&CK model for ICS environments, in order to correlate alerts and predict the risk of an on-going incident.
The information from these analyses will be considered for the context-aware classification of alerts.
Resultados
Publicaciones
Díaz-Verdejo, Jesús E.; Estepa Alonso, Rafael; Estepa Alonso, Antonio; Muñoz-Calle, F. J.; Madinabeitia, German
Building a large, realistic and labeled HTTP URI dataset for anomaly-based intrusion detection systems: Biblio-US17 Artículo de revista En preparación
En: Cybersecurity, En preparación, ISSN: 2523-3246.
@article{Biblio24,
title = {Building a large, realistic and labeled HTTP URI dataset for anomaly-based intrusion detection systems: Biblio-US17 },
author = {Jesús E. {Díaz-Verdejo} and {Estepa Alonso}, Rafael and {Estepa Alonso}, Antonio and F. J. Muñoz-Calle and German
Madinabeitia},
doi = {https://doi.org/10.1186/s42400‑024‑00336‑3},
issn = {2523-3246},
year = {2024},
date = {2024-12-11},
urldate = {2024-12-11},
journal = {Cybersecurity},
abstract = {This paper introduces Biblio-US17, a labeled dataset collected over 6 months from the log files of a popular public website at the University of Seville. It contains 47 million records, each including the method, uniform resource identifier (URI) and associated response code and size of every request received by the web server. Records have been classified as either normal or attack using a comprehensive semi-automated process, which involved signature-based detection, assisted inspection of URIs vocabulary, and substantial expert manual supervision. Unlike comparable datasets, this one offers a genuine real-world perspective on the normal operation of an active website, along with an unbiased proportion of actual attacks (i.e., non-synthetic). This makes it ideal for evaluating and comparing anomalybased approaches in a realistic environment. Its extensive size and duration also make it valuable for addressing challenges like data shift and insufficient training. This paper describes the collection and labeling processes, dataset structure, and most relevant properties. We also include an example of an application for assessing the performance of a simple anomaly detector. Biblio-US17, now available to the scientific community, can also be used to model the URIs used by current web servers.},
keywords = {},
pubstate = {forthcoming},
tppubtype = {article}
}
Lara, Agustín; Estepa, Antonio; Estepa, Rafael; Díaz-Verdejo, Jesús E.; Mayor, Vicente
Anomaly-based Intrusion Detection System for smart lighting Artículo de revista
En: Internet of Things, vol. 28, pp. 101427, 2024, ISSN: 2542-6605.
@article{LARA2024101427,
title = {Anomaly-based Intrusion Detection System for smart lighting},
author = {Agustín Lara and Antonio Estepa and Rafael Estepa and Jesús E. Díaz-Verdejo and Vicente Mayor},
url = {https://www.sciencedirect.com/science/article/pii/S2542660524003688},
doi = {https://doi.org/10.1016/j.iot.2024.101427},
issn = {2542-6605},
year = {2024},
date = {2024-01-01},
urldate = {2024-01-01},
journal = {Internet of Things},
volume = {28},
pages = {101427},
abstract = {Smart Lighting Systems (SLS) are essential to smart cities, offering enhanced energy efficiency and public safety. However, they are susceptible to security threats, potentially leading to safety risks and service disruptions, making the protection of this infrastructure critical. This paper presents an anomaly-based Intrusion Detection System (IDS) designed for a real-world operational SLS. As commercial deployments vary in components, protocols, and functionalities, IDSs must be tailored to the specific characteristics of each deployment to perform effectively. Our anomaly-based IDS has been defined based on the properties of the available data and the types of attacks we aim to detect, offering both explainability and low complexity. The proposed system identifies anomalies in seven features of network traffic and in the telemetry data received at the central control (O&M) server. For the latter, we designed three customized detectors to identify abnormal data points, persistent deviations in street lamp power consumption, and abnormal power value based on the time of day. Validation with real-world data and simulated attacks demonstrates the effectiveness of our approach. Network attacks (e.g., DoS, scanning) were detected by at least one of the seven flow-related anomaly detectors, while simulated data poisoning attacks and operational technology (OT) issues were detected with nearly 90% accuracy. The datasets used in this work are publicly available and may serve as reference for the design of future IDSs. While our detectors were designed specifically for our dataset, the variables examined and vulnerabilities addressed are common in most commercial SLSs.},
keywords = {},
pubstate = {published},
tppubtype = {article}
}
Muñoz-Calle, Javier; Alonso, Rafael Estepa; Alonso, Antonio Estepa; Díaz-Verdejo, Jesús E.; Fernández, Elvira Castillo; Madinabeitia, Germán
A Flexible Multilevel System for Mitre ATT&CK Model-driven Alerts and Events Correlation in Cyberattacks Detection Artículo de revista
En: JUCS – Journal of Universal Computer Science, vol. 30, no 9, pp. 1184-1204, 2024, ISSN: 0948-695X.
@article{10.3897/jucs.131686,
title = {A Flexible Multilevel System for Mitre ATT&CK Model-driven Alerts and Events Correlation in Cyberattacks Detection},
author = {Javier Muñoz-Calle and Rafael Estepa Alonso and Antonio Estepa Alonso and Jesús E. Díaz-Verdejo and Elvira Castillo Fernández and Germán Madinabeitia},
url = {https://doi.org/10.3897/jucs.131686},
doi = {10.3897/jucs.131686},
issn = {0948-695X},
year = {2024},
date = {2024-01-01},
urldate = {2024-01-01},
journal = {JUCS - Journal of Universal Computer Science},
volume = {30},
number = {9},
pages = {1184-1204},
publisher = {Journal of Universal Computer Science},
abstract = {Network monitoring systems can struggle to detect the full sequence of actions in a multi-step cyber attack, frequently resulting in multiple alerts (some of which are false positive (FP)) and missed actions. The challenge of easing the job of security analysts by triggering a single and accurate alert per attack requires developing and evaluating advanced event correlation techniques and models that have the potential to devise relationships between the different observed events/alerts.This work introduces a flexible architecture designed for hierarchical and iterative correlation of alerts and events. Its key feature is the sequential correlation of operations targeting specific attack episodes or aspects. This architecture utilizes IDS alerts or similar cybersecurity sensors, storing events and alerts in a non-relational database. Modules designed for knowledge creation then query these stored items to generate meta-alerts, also stored in the database. This approach facilitates creating a more refined knowledge that can be built on top of existing one by creating specialized modules. For illustrative purposes, we make a case study where we use this architectural approach to explore the feasibility of monitoring the progress of attacks of increased complexity by increasing the levels of the hyperalerts defined, including a case of a multi-step attack that adheres to the ATT&CK model. Although the mapping between the observations and the model components (i.e., techniques and tactics) is challenging, we could fully monitor the progress of two attacks and up to 5 out of 6 steps of the most complex attack by building up to three specialized modules. Despite some limitations due to the sensors and attack scenarios tested, the results indicate the architecture’s potential for enhancing the detection of complex cyber attacks, offering a promising direction for future cybersecurity research.},
keywords = {},
pubstate = {published},
tppubtype = {article}
}
Díaz-Verdejo, Jesús E.; Estepa Alonso, Rafael; Estepa Alonso, Antonio; Muñoz-Calle, Javier; Madinabeitia, Germán
Biblio-US17: A labeled real URL dataset for anomaly-based intrusion detection systems development Proceedings Article
En: European Interdisciplinary Cybersecurity Conference (EICC 2024), pp. 217–218, 2024, ISBN: 9798400716515.
@inproceedings{Diaz-Verdejo2024b,
title = {Biblio-US17: A labeled real URL dataset for anomaly-based intrusion detection systems development},
author = {Jesús E. Díaz-Verdejo and {Estepa Alonso}, Rafael and {Estepa Alonso}, Antonio and Javier Muñoz-Calle and Germán Madinabeitia},
doi = {10.1145/3655693.3661319},
isbn = {9798400716515},
year = {2024},
date = {2024-01-01},
urldate = {2024-01-01},
booktitle = {European Interdisciplinary Cybersecurity Conference (EICC 2024)},
pages = {217–218},
abstract = {The development of anomaly-based intrusion detection systems is hindered by the scarcity of adequate datasets. An ideal dataset should contain real traffic, genuine attacks and cover a large time period that may demonstrate time shift. To be useful, the dataset must be labeled to provide accurate ground-truth, This paper presents a dataset of URLs that possesses these qualities. It can therefore be used to effectively train, test, and validate URL-based anomaly detection systems. The dataset is publicly available and contains 47M registers, including 320k attacks, and spans for 6.5 months. It is partitioned acording to two schemes to allow for time dependent and time independent experiments.},
keywords = {},
pubstate = {published},
tppubtype = {inproceedings}
}
Díaz-Verdejo, J.; Alonso, R. Estepa; Alonso, A. Estepa; Muñoz-Calle, F. J.
Impacto de la evolución temporal de datasets reales en el rendimiento de un IDS basados en anomalías: estudio experimental sobre HTTP Proceedings Article
En: XI Jornadas Nacionales de Investigación en Ciberseguridad, pp. 302–309, 2024.
@inproceedings{DiazVerdejo2024,
title = {Impacto de la evolución temporal de datasets reales en el rendimiento de un IDS basados en anomalías: estudio experimental sobre HTTP},
author = {J. Díaz-Verdejo and R. Estepa Alonso and A. Estepa Alonso and F. J. Muñoz-Calle},
year = {2024},
date = {2024-01-01},
urldate = {2024-01-01},
booktitle = {XI Jornadas Nacionales de Investigación en Ciberseguridad},
pages = {302–309},
abstract = {El desarrollo y evaluación de sistemas de detección de intrusiones basados en anomalías es de vital importancia en el contexto de la ciberseguridad, especialmente en relación a los ataques de día cero. La naturaleza altamente diamica tanto de los sistemas a proteger como de los ataques hace que la detección de anomalías resulte una tarea compleja, ya que esta evolución temporal puede afectar a las capacidades de los modelos estimados en un escenario y periodo determinados. A pesar de su importancia, este efecto ha sido explorado de forma limitada en la literatura, especialmente por la prática inexistencia de datos reales convenientemente etiquetados con la suficiente extensión temporal. En el presente trabajo evaluamos experimentalmente el impacto de la evolución temporal en un sistema para la detección de ataques basados en URL utilizando datos reales capturados en un escenario real durante un periodo de tiempo relativamente extenso. Nuestros análisis demuestran una degradación de creciente con la distancia temporal entre el entrenamiento y la evaluación. Esta degradación es debida a la combinación de la pérdida de calidad del modelo con el tiempo así como a la propia variación del comportamiento del servicio y/o ataques a lo largo del tiempo.},
keywords = {},
pubstate = {published},
tppubtype = {inproceedings}
}
Díaz-Verdejo, J.; Muñoz-Calle, J.; Alonso, R. Estepa; Alonso, A. Estepa
InspectorLog : A New Tool for Offline Attack Detection over Web Log Proceedings Article
En: Proceedings of the 21st International Conference on Security and Cryptography (SECRYPT 2024), pp. 692–697, 2024, ISBN: 9789897587092.
@inproceedings{Diaz-Verdejo2024a,
title = {InspectorLog : A New Tool for Offline Attack Detection over Web Log},
author = {J. Díaz-Verdejo and J. Muñoz-Calle and R. Estepa Alonso and A. Estepa Alonso},
doi = {10.5220/0012764000003767},
isbn = {9789897587092},
year = {2024},
date = {2024-01-01},
urldate = {2024-01-01},
booktitle = {Proceedings of the 21st International Conference on Security and Cryptography (SECRYPT 2024)},
number = {Secrypt},
pages = {692–697},
abstract = {InspectorLog is a novel tool for offline analysis of HTTP logs. The tool processes web server logs to identify attacks using diverse rule sets, focusing primarily on the URI field. It is compatible with standard rule formats from systems such as Snort, Nemesida, and ModSecurity. This paper describes InspectorLog functionalities, architecture and applications to the scientific community. We also experimentally validate InspectorLog by comparing its detection power with that of the IDS from which rules are taken. Inspector log fills a gap in available tools in cybersecurity practices in forensic analysis, dataset sanitization, and signature tuning. Future enhancements are planned to support additionalWeb Application Firewalls (WAFs), new rule types, and HTTP protocol methods, aiming to broaden its scope and utility in the ever-evolving domain of network security.},
keywords = {},
pubstate = {published},
tppubtype = {inproceedings}
}
Díaz-Verdejo, Jesús; Alonso, Rafael Estepa; Alonso, Antonio Estepa; Muñoz-Calle, Javier
Insights into anomaly-based intrusion detection systems usability. A case study using real http requests Proceedings Article
En: Proc. European Interdisciplinary Cybersecurity Conference (EICC 2024), pp. 82–89, 2024, ISBN: 9798400716515.
@inproceedings{Diaz-Verdejo2024,
title = {Insights into anomaly-based intrusion detection systems usability. A case study using real http requests},
author = {Jesús Díaz-Verdejo and Rafael Estepa Alonso and Antonio Estepa Alonso and Javier Muñoz-Calle},
doi = {10.1145/3655693.3655745},
isbn = {9798400716515},
year = {2024},
date = {2024-01-01},
urldate = {2024-01-01},
booktitle = {Proc. European Interdisciplinary Cybersecurity Conference (EICC 2024)},
pages = {82–89},
abstract = {Intrusion detection systems based on anomalies (A-IDS) are crucial for detecting cyberattacks, especially zero-day attacks. Numerous A-IDS proposals in the literature report excellent performance according to established metrics and settings in a laboratory. However, finding systems implementing these proposals in real-world scenarios is challenging. This work explores, through a case study, the suitability of performance metrics commonly used in the scientific literature to real-world scenarios. Our case study will consider a Web attack detector based on URIs and a real, large-scale dataset. Our results show significant limitations in the performance metrics commonly used to select the system's operating point and its practical use in real-world scenarios.},
keywords = {},
pubstate = {published},
tppubtype = {inproceedings}
}
Walabonso Lara, Agustín; Mayor, Vicente; Estepa Alonso, Rafael; Estepa Alonso, Antonio; Díaz-Verdejo, Jesús E.
Smart home anomaly-based IDS: Architecture proposal and case study Artículo de revista
En: Internet of Things, vol. 22, pp. 100773, 2023, ISSN: 2542-6605.
@article{Lara2023,
title = {Smart home anomaly-based IDS: Architecture proposal and case study},
author = { {Walabonso Lara}, Agustín and Vicente Mayor and {Estepa Alonso}, Rafael and {Estepa Alonso} , Antonio and Jesús E. {Díaz-Verdejo}},
url = {https://linkinghub.elsevier.com/retrieve/pii/S2542660523000963},
doi = {10.1016/J.IOT.2023.100773},
issn = {2542-6605},
year = {2023},
date = {2023-07-01},
urldate = {2023-07-01},
journal = {Internet of Things},
volume = {22},
pages = {100773},
publisher = {Elsevier},
abstract = {The complexity and diversity of the technologies involved in the Internet of Things (IoT) challenge the generalization of security solutions based on anomaly detection, which should fit the particularities of each context and deployment and allow for performance comparison. In this work, we provide a flexible architecture based on building blocks suited for detecting anomalies in the network traffic and the application-layer data exchanged by IoT devices in the context of Smart Home. Following this architecture, we have defined a particular Intrusion Detector System (IDS) for a case study that uses a public dataset with the electrical consumption of 21 home devices over one year. In particular, we have defined ten Indicators of Compromise (IoC) to detect network attacks and two anomaly detectors to detect false command or data injection attacks. We have also included a signature-based IDS (Snort) to extend the detection range to known attacks. We have reproduced eight network attacks (e.g., DoS, scanning) and four False Command or Data Injection attacks to test our IDS performance. The results show that all attacks were successfully detected by our IoCs and anomaly detectors with a false positive rate lower than 0.3%. Signature detection was able to detect only 4 out of 12 attacks. Our architecture and the IDS developed can be a reference for developing future IDS suited to different contexts or use cases. Given that we use a public dataset, our contribution can also serve as a baseline for comparison with new techniques that improve detection performance.},
keywords = {},
pubstate = {published},
tppubtype = {article}
}
Castillo-Fernández, Elvira; Muñoz, Escolástico; Diaz-Verdejo, J.; Estepa Alonso, R; Estepa Alonso, A.
Diseño y despliegue de un laboratorio para formación e investigación en ciberseguridad Proceedings Article
En: Actas de las VIII Jornadas Nacionales de Investigación en Ciberseguridad (JNIC23) , pp. 445-452, 2023, ISBN: 978-84-8158-970-2.
@inproceedings{jnic23-cslab,
title = {Diseño y despliegue de un laboratorio para formación e investigación en ciberseguridad},
author = {Elvira Castillo-Fernández and Escolástico Muñoz and J. Diaz-Verdejo and {Estepa Alonso}, R and {Estepa Alonso}, A.},
isbn = {978-84-8158-970-2},
year = {2023},
date = {2023-06-21},
urldate = {2023-06-21},
booktitle = {Actas de las VIII Jornadas Nacionales de Investigación en Ciberseguridad (JNIC23) },
journal = {Actas de las VIII Jornadas Nacionales de Investigación en Ciberseguridad (JNIC23) - En revisión},
pages = {445-452},
abstract = {La realización de simulacros y/o experimentos para actividades de formación e investigación en ciberseguridad plantea serias dificultades prácticas por la ejecución de ataques a los sistemas que conforman la propia infraestructura. Se presentan múltiples requisitos, en ocasiones, incompatibles entre sí, como la necesidad de preservar la seguridad de los sistemas externos y de monitorización sin perder la conectividad hacia Internet, la capacidad de monitorización y adquisición de trazas de una forma segura, la flexibilidad que permita múltiples escenarios lo más realistas posible y una fácil reusabilidad del laboratorio. En el presente trabajo se propone e implementa una arquitectura para un laboratorio de ciberseguridad que presenta un equilibrio entre flexibilidad, funcionalidad, usabilidad y seguridad de las operaciones. La propuesta se basa en la división en una red de supervisión y una red de laboratorio sobre la que, mediante virtualización de bajo nivel, se pueden desarrollar los diferentes experimentos y ataques con riesgo mínimo de impacto sobre la red de supervisión. Para ello se establecen diferentes barreras, tanto físicas como lógicas, que permiten filtrar el tráfico entre ambas y la conectividad hacia Internet. Para mostrar la operación y capacidades de la arquitectura propuesta se presenta un caso de uso con un ataque multietapa que involucra diversos sistemas operativos y equipos.},
keywords = {},
pubstate = {published},
tppubtype = {inproceedings}
}
Castillo-Fernández, E.; Diaz-Verdejo, J.; Estepa Alonso, R.; Estepa Alonso, A.
Riesgos en la Smart Home: estudio experimental Proceedings Article
En: Actas de las VIII Jornadas Nacionales de Investigación en Ciberseguridad (JNIC23), pp. 375-382, 2023, ISBN: 978-84-8158-970-2.
@inproceedings{jnic23-iot,
title = {Riesgos en la Smart Home: estudio experimental},
author = {E. Castillo-Fernández and J. Diaz-Verdejo and {Estepa Alonso}, R. and {Estepa Alonso}, A.},
isbn = {978-84-8158-970-2},
year = {2023},
date = {2023-06-21},
urldate = {2023-06-21},
booktitle = {Actas de las VIII Jornadas Nacionales de Investigación en Ciberseguridad (JNIC23)},
pages = {375-382},
abstract = {En este trabajo realizamos una evaluación preliminar de los riesgos de ciberseguridad en un escenario de aplicación típico de SmartHome: una vivienda unifamiliar. Para ello se han desplegado varias tecnologías comúnmente utilizadas en este contexto y se ha monitorizado el tráfico asociado a los dispositivos y servidores SmartHome. A partir del análisis realizado se ha constatado la existencia de ataques, patrones de comunicación anómalos entre dispositivos y con servidores externos, así como vulnerabilidades asociadas a debilidades en las configuraciones de los dispositivos y los protocolos desplegados, algunos de ellos propietarios. Adicionalmente, para algunos dispositivos se ha constatado una gran dependencia de la nube, lo que facilita la indisponibilidad de algunos servicios en caso de fallos en la conexión con nube. El resultado evidencia un pobre tratamiento de la ciberseguridad por la mayoría de los operadores del sector y un riesgo en este tipo de instalaciones que puede pasar inadvertido al usuario.},
keywords = {},
pubstate = {published},
tppubtype = {inproceedings}
}
Lara, Agustín W.; Ternero, J. A.; Estepa Alonso, Rafael; Estepa Alonso, Antonio; Ruiz-Robles, Fernando; Díaz-Verdejo, Jesús E.
HTTP Cyberattacks Detection through Automatic Signature Generation in multi-site IoT Deployments Proceedings Article
En: Proc. European Interdisciplinary Cybersecurity Conference (EICC 2023) , pp. 6, 2023.
@inproceedings{eicc2-firmas,
title = {HTTP Cyberattacks Detection through Automatic Signature Generation in multi-site IoT Deployments},
author = {Agustín W. Lara and J.A. Ternero and {Estepa Alonso}, Rafael and {Estepa Alonso}, Antonio and Fernando Ruiz-Robles and Jesús E. Díaz-Verdejo
},
doi = {10.1145/3590777.3590788},
year = {2023},
date = {2023-06-14},
urldate = {2023-06-14},
booktitle = {Proc. European Interdisciplinary Cybersecurity Conference (EICC 2023)
},
pages = {6},
abstract = { IoT deployments often include a web-interface server for managerial purposes. Signature-based Intrusion Detection Systems are commonly used to detect HTTP attacks on these web servers. The standard signature repositories used by these defensive systems can be enhanced with new signatures generated automatically from attacks detected with anomaly detection techniques.
This work presents a scheme for generating such anomaly-based signatures from HTTP attacks in a way that avoids excessive false positives. The signatures generated are distributed to peer sites in a multi-site environment. We also present a case study based on an IoT real-life dataset collected at four different SmartLight deployments from the same organization. Our results show a notable performance improvement (from $24.1%$ to $66.7%$) when anomaly-based signatures are added to the standard default Snort ruleset and distributed to the other three sites.},
keywords = {},
pubstate = {published},
tppubtype = {inproceedings}
}
This work presents a scheme for generating such anomaly-based signatures from HTTP attacks in a way that avoids excessive false positives. The signatures generated are distributed to peer sites in a multi-site environment. We also present a case study based on an IoT real-life dataset collected at four different SmartLight deployments from the same organization. Our results show a notable performance improvement (from $24.1%$ to $66.7%$) when anomaly-based signatures are added to the standard default Snort ruleset and distributed to the other three sites.
Fernández, Elvira Castillo; Díaz-Verdejo, Jesús E.; Estepa Alonso, Rafael; Estepa Alonso, Antonio; Muñoz-Calle, Javier; Madinabeitia, Germán
Multistep Cyberattacks Detection using a Flexible Multilevel System for Alerts and Events Correlation Proceedings Article
En: Proc. European Interdisciplinary Cybersecurity Conference (EICC 2023), pp. 6, 2023.
@inproceedings{eicc23-attacks,
title = {Multistep Cyberattacks Detection using a Flexible Multilevel System for Alerts and Events Correlation},
author = {Elvira {Castillo Fernández} and Jesús E. {Díaz-Verdejo} and {Estepa Alonso}, Rafael and {Estepa Alonso}, Antonio and Javier {Muñoz-Calle} and Germán Madinabeitia},
doi = {10.1145/3590777.3590778},
year = {2023},
date = {2023-06-14},
urldate = {2023-06-14},
booktitle = {Proc. European Interdisciplinary Cybersecurity Conference (EICC 2023)},
pages = {6},
abstract = {Current network monitoring systems tend to generate several alerts per attack, especially in multistep attacks. However, Cybersecurity Officers (CSO) would rather receive a single alert summarizing the entire incident. Triggering a single alert per attack is a challenge that requires developing and evaluating advanced event correlation techniques and models to determine the relationships between the different observed events/alerts.
In this work, we propose a flexible architecture oriented toward the correlation and aggregation of events and alerts in a multilevel iterative approach.
In our scheme, sensors generate events and alerts that are stored in a non-relational database queried by modules that create knowledge structured as meta-alerts that are also stored in the database. These meta-alerts (also called hyperalerts) are, in turn, used iteratively to create new knowledge. This iterative approach can be used to aggregate information at multiple levels or steps in complex attack models.
Our architecture also allows the incorporation of additional sensors and the evaluation of various correlation techniques and multistage attack models. The capabilities of the system are assessed through three case studies.},
keywords = {},
pubstate = {published},
tppubtype = {inproceedings}
}
In this work, we propose a flexible architecture oriented toward the correlation and aggregation of events and alerts in a multilevel iterative approach.
In our scheme, sensors generate events and alerts that are stored in a non-relational database queried by modules that create knowledge structured as meta-alerts that are also stored in the database. These meta-alerts (also called hyperalerts) are, in turn, used iteratively to create new knowledge. This iterative approach can be used to aggregate information at multiple levels or steps in complex attack models.
Our architecture also allows the incorporation of additional sensors and the evaluation of various correlation techniques and multistage attack models. The capabilities of the system are assessed through three case studies.
Castillo-Fernández, Elvira; Díaz-Verdejo, Jesús Esteban; Alonso, Rafael María Estepa; Alonso, Antonio Estepa; Muñoz-Calle, Fco Javier
Uso practico del modelo ATT&CK para la detección de ciberataques Proceedings Article
En: Actas de las XVI Jornadas de Ingeniería Telemática – JITEL 2023, pp. 1–4, 2023, ISBN: 9783131450715.
@inproceedings{Castillo-Fernandez2023,
title = {Uso practico del modelo ATT&CK para la detección de ciberataques},
author = {Elvira Castillo-Fernández and Jesús Esteban Díaz-Verdejo and Rafael María Estepa Alonso and Antonio Estepa Alonso and Fco Javier Muñoz-Calle},
isbn = {9783131450715},
year = {2023},
date = {2023-01-01},
urldate = {2023-01-01},
booktitle = {Actas de las XVI Jornadas de Ingeniería Telemática - JITEL 2023},
pages = {1–4},
abstract = {ATT&CK establece un modelo donde se especifican las fases secuenciales de un ciberataque, así como las técnicas que suelen ser usadas en cada paso del ataque. Sería interesante incorporar este modelo en el proceso de detección de los ciberataques ya que facilitaría la correlación de las numerosas alertas generadas por los sistemas de monitorización de red. Sin embargo, la aplicación del modelo en los procesos de correlación de eventos no es inmediata, ya que no está formulado en términos de eventos observables y/o detecciones sino de acciones a realizar. En el presente trabajo exploramos y evaluamos los elementos necesarios para incorporar el modelo ATT&CK en el procesamiento de la información generada por los sistemas de monitorización de la seguridad en la red.},
keywords = {},
pubstate = {published},
tppubtype = {inproceedings}
}
Muñoz-calle, Javier; Fructuoso, Javier; Estepa, Rafael; Estepa, Antonio
Evaluación experimental de las capacidades de detección de ciberataques basados en técnicas del modelo ATT & CK mediante Snort Proceedings Article
En: Actas de las XVI Jornadas de Ingeniería Telemática – JITEL 2023, pp. 5–8, 2023.
@inproceedings{Munoz-calle2023,
title = {Evaluación experimental de las capacidades de detección de ciberataques basados en técnicas del modelo ATT & CK mediante Snort},
author = {Javier Muñoz-calle and Javier Fructuoso and Rafael Estepa and Antonio Estepa},
year = {2023},
date = {2023-01-01},
urldate = {2023-01-01},
booktitle = {Actas de las XVI Jornadas de Ingeniería Telemática - JITEL 2023},
pages = {5–8},
abstract = {ATT&CK establece un modelo donde se especifican las fases secuenciales de un ciberataque, as´ı como las t´ecnicas que suelen ser usadas en cada paso del ataque. Ser´ıa interesante incorporar este modelo en el proceso de detecci´on de los ciberataques ya que facilitar´ıa la correlaci´on de las numerosas alertas generadas por los sistemas de monitorizaci´on de red. Sin embargo, la aplicaci´on del modelo en los procesos de correlaci´on de eventos no es inmediata, ya que no est´a formulado en t´erminos de eventos observables y/o detecciones sino de acciones a realizar. En el presente trabajo exploramos y evaluamos los elementos necesarios para incorporar el modelo ATT&CK en el procesamiento de la informaci´on generada por los sistemas de monitorizaci´on de la seguridad en la red.},
keywords = {},
pubstate = {published},
tppubtype = {inproceedings}
}
Díaz-Verdejo, Jesús E.; Estepa Alonso, Rafael; Estepa Alonso, Antonio; Madinabeitia, German
A critical review of the techniques used for anomaly detection of HTTP-based attacks: taxonomy, limitations and open challenges Artículo de revista
En: Computers and Security, vol. 124, pp. 102997, 2023, ISSN: 01674048.
@article{Diaz-Verdejo2023,
title = {A critical review of the techniques used for anomaly detection of HTTP-based attacks: taxonomy, limitations and open challenges},
author = {Jesús E. Díaz-Verdejo and {Estepa Alonso}, Rafael and {Estepa Alonso}, Antonio and German Madinabeitia},
doi = {10.1016/j.cose.2022.102997},
issn = {01674048},
year = {2023},
date = {2023-01-01},
urldate = {2023-01-01},
journal = {Computers and Security},
volume = {124},
pages = {102997},
abstract = {Intrusion Detection Systems (IDSs) and Web Application Firewalls (WAFs) offer a crucial layer of defense that allows organizations to detect cyberattacks on their web servers. Academic research overwhelmingly suggests using anomaly detection techniques to improve the performance of these defensive systems. However, analyzing and comparing the wide range of solutions in the scientific literature is challenging since they are typically presented as isolated (unrelated) contributions, and their results cannot be generalized. We believe that this impairs the industry's adoption of academic results and the advancement of research in this field. This paper aims to shed light on the literature on anomaly-based detection of attacks that use HTTP request messages. We define a novel framework for anomaly detection based on six data processing steps grouped into two sequential phases: preprocessing and classification. Based on this framework, we provide a taxonomy and critical review of the techniques surveyed, emphasizing their limitations and applicability. Future approaches should take advantage of the syntax and semantics of the Uniform Resource Locator (URL), be scalable, and address their obsolescence. These aspects are frequently overlooked in the literature and pose a significant challenge in the current era of web services. For better comparability, authors should use adequate public datasets, follow a thorough methodology, and use appropriate metrics that fully show the pros and cons of the approach.},
keywords = {},
pubstate = {published},
tppubtype = {article}
}
Muñoz, Javier; Bueno, Felipe; Estepa, Rafael; Estepa, Antonio; Díaz-Verdejo, Jesús E.
Ataques a servidores web: estudio experimental de la capacidad de detección de algunos SIDS gratuitos Proceedings Article
En: Actas de las VII Jornadas Nacionales de Investigación en Ciberseguridad (JNIC'22), pp. 22–25, 2022, ISBN: 9878488734136.
@inproceedings{Munoz-jnic22,
title = {Ataques a servidores web: estudio experimental de la capacidad de detección de algunos SIDS gratuitos},
author = {Javier Muñoz and Felipe Bueno and Rafael Estepa and Antonio Estepa and Jesús E. Díaz-Verdejo},
isbn = {9878488734136},
year = {2022},
date = {2022-01-01},
urldate = {2022-01-01},
booktitle = {Actas de las VII Jornadas Nacionales de Investigación en Ciberseguridad (JNIC'22)},
pages = {22--25},
abstract = {Este trabajo cuantifica de forma experimental la capacidad de detección de ataques a servidores web ofrecida por algunos de los detectores de intrusiones basados en firmas (SIDS) disponibles de forma gratuita. Para ello, se ha realizado una búsqueda y selección de 28 herramientas actuales para la generación de ataques y análisis de seguridad del servicio web. Con ellas, se han realizado casi 150 ataques a dos escenarios de uso de un servidor web (una web estática y una dinámica). Las peticiones HTTP registradas durante los ataques han sido utilizadas para crear un dataset de ataques que será utilizado como entrada a tres SIDS gratuitos seleccionados por su amplio uso, de forma que se podrá determinar la capacidad de detección de los mismos frente a los ataques generados. Este trabajo se encuentra aún en desarrollo, por lo que en esta contribución se muestran los primeros resultados relativos a la recolección y selección de herramientas para la generación de los ataques, la generación del dataset de ataques de forma que sea representativo de los ataques actuales y la evaluación preliminar de las capacidades de detección.},
keywords = {},
pubstate = {published},
tppubtype = {inproceedings}
}
Díaz-Verdejo, J. E.; Muñoz-Calle, F. J.; Estepa Alonso, A.; Estepa Alonso, R.; Madinabeitia, G.
On the Detection Capabilities of Signature-Based Intrusion Detection Systems in the Context of Web Attacks Artículo de revista
En: Applied Sciences, vol. 12, no 2, pp. 852, 2022, ISSN: 20763417.
@article{Diaz-Verdejo2022,
title = {On the Detection Capabilities of Signature-Based Intrusion Detection Systems in the Context of Web Attacks},
author = {J. E. Díaz-Verdejo and F. J. Muñoz-Calle and {Estepa Alonso}, A. and {Estepa Alonso}, R. and G. Madinabeitia},
url = {https://www.mdpi.com/2076-3417/12/2/852/htm https://www.mdpi.com/2076-3417/12/2/852},
doi = {10.3390/app12020852},
issn = {20763417},
year = {2022},
date = {2022-01-01},
urldate = {2022-01-01},
journal = {Applied Sciences},
volume = {12},
number = {2},
pages = {852},
publisher = {Multidisciplinary Digital Publishing Institute},
abstract = {Signature-based Intrusion Detection Systems (SIDS) play a crucial role within the arsenal of security components of most organizations. They can find traces of known attacks in the network traffic or host events for which patterns or signatures have been pre-established. SIDS include standard packages of detection rulesets, but only those rules suited to the operational environment should be activated for optimal performance. However, some organizations might skip this tuning process and instead activate default off-the-shelf rulesets without understanding its implications and trade-offs. In this work, we help gain insight into the consequences of using predefined rulesets in the performance of SIDS. We experimentally explore the performance of three SIDS in the context of web attacks. In particular, we gauge the detection rate obtained with predefined subsets of rules for Snort, ModSecurity and Nemesida using seven attack datasets. We also determine the precision and rate of alert generated by each detector in a real-life case using a large trace from a public webserver. Results show that the maximum detection rate achieved by the SIDS under test is insufficient to protect systems effectively and is lower than expected for known attacks. Our results also indicate that the choice of predefined settings activated on each detector strongly influences its detection capability and false alarm rate. Snort and ModSecurity scored either a very poor detection rate (activating the less-sensitive predefined ruleset) or a very poor precision (activating the full ruleset). We also found that using various SIDS for a cooperative decision can improve the precision or the detection rate, but not both. Consequently, it is necessary to reflect upon the role of these open-source SIDS with default configurations as core elements for protection in the context of web attacks. Finally, we provide an efficient method for systematically determining which rules deactivate from a ruleset to significantly reduce the false alarm rate for a target operational environment. We tested our approach using Snort’s ruleset in our real-life trace, increasing the precision from 0.015 to 1 in less than 16 h of work.},
keywords = {},
pubstate = {published},
tppubtype = {article}
}
Datos
- Biblio-US17 – Base de datos de peticiones HTTP reales etiquetada (42 M de registros) Más información
- IoT SmartHome – Dataset real de tráfico en Smart HomeMás información
- IoT SmartLighting – Dataset real de tráfico en despliegue Smart Lighting Más información
Software / sistemas
- Monitorización red Smart Home – Red para la monitorización y captura de tráfico real en Smart Home Más información
- Inspectorlog – Herramienta de análisis de trazas HTTP basada en firmas Más información
- NE-SIEM – Prototipo de sistema integral de detección con capacidad multifuente y multiplanta
- Laboratorio ciberseguridad – Laboratorio híbrido orientado a la experimentación y docencia en ciberseguridad Más información